Fabio Poiesi
I'm the Head of the Technologies of Vision Lab at Fondazione Bruno Kessler in Trento, Italy.
I work on computer vision for both research and real-world industrial applications.
I did my PhD and Postdoc at Queen Mary University of London with Andrea Cavallaro as my supervisor.
There, I worked on algorithms for multi-object tracking, target behaviour understanding, and vision-based quadrotor manoeuvring.
I joined FBK in 2016 as a Research Scientist and became a tenured researcher in 2023.
At FBK, I started working on 3D scene understanding and have been doing research in that area, as well as in 2D scene understanding.
Below is a selection of my scholarly publications.
Email /
Google Scholar /
X /
Linkedin /
github
|
|
|
Unsupervised Point Cloud Representation Learning by Clustering and Neural Rendering
Guofeng Mei,
Cristiano Saltori,
Elisa Ricci,
Nicu Sebe,
Qiang Wu,
Jian Zhang,
Fabio Poiesi
International Journal of Computer Vision (IJCV), Mar 2024
Augmentation-free unsupervised approach for point clouds to learn transferable point-level features by leveraging uni-modal information for soft clustering and cross-modal information for neural rendering.
|
|
Compositional Semantic Mix for Domain Adaptation in Point Cloud Segmentation
Cristiano Saltori,
Fabio Galasso,
Giuseppe Fiameni,
Nicu Sebe,
Fabio Poiesi,
Elisa Ricci
IEEE Trans. on Pattern Analysis and Machine Intelligence (TPAMI), Dec 2023
Compositional semantic mixing for point cloud domain adaptation, representing the first unsupervised domain adaptation technique for point cloud segmentation based on semantic and geometric sample mixing.
|
|
Survey on video anomaly detection in dynamic scenes with moving cameras
Runyu Jiao,
Yi Wan,
Fabio Poiesi,
Yiming Wang
Artificial Intelligence Review, Oct 2023
First comprehensive survey on Moving Camera Video Anomaly Detection (MC-VAD).
We delve into the research papers related to MC-VAD, critically assessing their limitations and highlighting associated challenges.
|
|
PatchMixer: Rethinking network design to boost generalization for 3D point cloud understanding
Davide Boscaini,
Fabio Poiesi
Image and Vision Computing (IMAVIS), Sep 2023 (github)
Simple yet effective architecture that extends the ideas behind MLP-Mixer to 3D point clouds. We process local patches instead of the whole shape to promote robustness to partial point clouds.
|
|
Attentive Multimodal Fusion for Optical and Scene Flow
Youjie Zhou,
Guofeng Mei,
Yiming Wang,
Fabio Poiesi,
Yi Wan
IEEE Robotics and Automation Letters (RAL), Jul 2023 (github)
Deep neural network to estimate optical flow based on early-stage information fusion between sensor modalities (RGB and depth).
We incorporate self- and cross-attention layers at different network levels to construct informative features that leverage the strengths of both modalities.
|
|
Exploiting multi-granularity visual features for retinal layer segmentation in human eyes
Xiang He,
Yiming Wang,
Fabio Poiesi,
Weiye Song,
Quanqing Xu,
Zixuan Feng,
Yi Wan
Frontiers in Bioengineering and Biotechnology, Jun 2023 (github)
End-to-end retinal layer segmentation network based on ConvNeXt that can retain more feature map details by using a new depth-efficient attention module and multiscale structures.
|
|
Learning general and distinctive 3D local deep descriptors for point cloud registration
Fabio Poiesi,
Davide Boscaini
IEEE Trans. on Pattern Analysis and Machine Intelligence (TPAMI), Mar 2023 (github)
Method to learn general and distinctive 3D local descriptors that can be used to register point clouds that are captured in different domains.
|
|
Loop closure detection using local 3D deep descriptors
Youjie Zhou,
Yiming Wang,
Fabio Poiesi,
Qi Qin,
Yi Wan
IEEE Robotics and Automation Letters (RAL), Jul 2022
Simple yet effective method to address loop closure detection in simultaneous localisation and mapping using local 3D deep descriptors (L3Ds). L3Ds are emerging com-
pact representations of patches extracted from point clouds that are learnt from data using a deep learning algorithm.
|
|
Performance comparison of image enhancers with and without deep learning
Michela Lecca,
Fabio Poiesi
Journal of the Optical Society of America A (JOSA), Apr 2022
We empirically compare a set of traditional and deep learning enhancers, which we selected as representing different methodologies for the improvement of bad illuminated images.
|
|
Multi-view data capture for dynamic object reconstruction using handheld augmented reality mobiles
Matteo Bortolon,
Luca Bazzanella,
Fabio Poiesi
Journal of Real-Time Image Processing (JRTIP), Mar 2021 (github)
System to capture nearlysynchronous frame streams from multiple and moving handheld mobiles that is suitable for dynamic object 3D reconstruction.
Each mobile executes Simultaneous Localisation and Mapping on-board to estimate its pose, and uses a wireless communication channel to send or receive synchronisation triggers.
|
|
Support Vector Motion Clustering
Isah A. Lawal,
Fabio Poiesi,
Davide Anguita,
Andrea Cavallaro
IEEE Trans. on Circuits and Systems for Video Technology (TCSVT), Nov 2017
Closed-loop unsupervised clustering method for motion vectors extracted from highly dynamic video scenes.
Motion vectors are assigned to non-convex homogeneous clusters characterizing direction, size and shape of regions with multiple independent activities.
|
|
Tracking multiple high-density homogeneous targets
Fabio Poiesi,
Andrea Cavallaro
IEEE Trans. on Circuits and Systems for Video Technology (TCSVT), Apr 2015 (video)
Framework for multi-target detection and tracking that infers candidate target locations in videos containing a high density of homogeneous targets.
|
|
Predicting and recognizing human interactions in public spaces
Fabio Poiesi,
Andrea Cavallaro
Journal on Real-Time Image Processing (JRTIP), Dec 2015
Method for predicting rendezvous areas in observable and unobservable regions using sparse motion information.
Rendezvous areas indicate where people are likely to interact with each other or with static objects (e.g. a door, an information desk or a meeting point).
|
|
Measures of effective video tracking
Tahir Nawaz,
Fabio Poiesi,
Andrea Cavallaro
IEEE Trans. on Image Processing (TIP), Jan 2014 (video)
Three parameter-independent measures for evaluating multi-target video tracking.
The measures take into account target-size variations, combine accuracy and cardinality errors, quantify long-term tracking accuracy at different accuracy levels, and evaluate ID changes relative to the duration of the track in which they occur.
|
|
Multi-target tracking on confidence maps: an application to people tracking
Fabio Poiesi,
Riccardo Mazzon,
Andrea Cavallaro
Computer Vision and Image Understanding (CVIU), Oct 2013 (video)
Generic online multi-target track-before-detect based on particle filtering that is applicable on confidence maps used as observations.
We include the target ID into the particle state, enabling tracking with unknown and large number of targets.
|
|
Open-vocabulary object 6D pose estimation
Jaime Corsetti,
Davide Boscaini,
Changjae Oh,
Andrea Cavallaro,
Fabio Poiesi
Computer Vision and Pattern Recognition (CVPR Highlight), Jun 2024 (website)
Our approach leverages a Vision-Language Model to segment the object of interest from two distinct scenes and to estimate its relative 6D pose.
We assume (i) the object of interest is specified solely through the textual prompt, (ii) no object model (e.g. CAD or video sequence) is required at inference, (iii) the object is imaged from two different viewpoints of two different scenes, and (iv) the object was not observed during the training phase.
|
|
Geometrically-driven aggregation for zero-shot 3D point cloud understanding
Guofeng Mei,
Luigi Riz,
Yiming Wang,
Fabio Poiesi
Computer Vision and Pattern Recognition (CVPR Highlight), Jun 2024 (website)
Training-free aggregation technique that leverages the point cloud's 3D geometric structure to improve the quality of the transferred Vision-Language Model representations.
Our approach operates iteratively, performing local-to-global aggregation based on geometric and semantic point-level reasoning.
|
|
IFFNeRF: Initialisation Free and Fast 6DoF pose estimation from a single image and a NeRF model
Matteo Bortolon,
Theodore Tsesmelis,
Stuart James,
Fabio Poiesi,
Alessio Del Bue
International Conference on Robotics and Automation (ICRA), May 2024 (website)
From a given image with an unknown pose and a NeRF model, we recover the pose by first sampling surface points using Metropolis-Hasting algorithm and casting rays from them in isocell distribution.
We then correlate rays with the image to identify relevant rays using attention and recover the unknown camera pose.
|
|
Detect, Augment, Compose, and Adapt: Four Steps for Unsupervised Domain Adaptation in Object Detection
Mohamed L. Mekhalfi,
Davide Boscaini,
Fabio Poiesi
British Machine Vision Conference (BMVC), Nov 2023 (github)
Effective four-step unsupervised domain adaptation approach that leverages self-supervision and trains source and target data concurrently.
|
|
Revisiting Fully Convolutional Geometric Features for Object 6D Pose Estimation
Jaime Corsetti,
Davide Boscaini,
Fabio Poiesi
International Conference on Computer Vision Workshops (ICCVW), Oct 2023 (github)
Revisiting Fully Convolutional Geometric Features (FCGF) to achieve object 6D pose estimation state-of-the-art performance.
FCGF employs sparse convolutions and learns point-level features using a fully-convolutional network by optimising a hardest contrastive loss.
|
|
Novel Class Discovery for 3D Point Cloud Semantic Segmentation
Luigi Riz,
Cristiano Saltori,
Elisa Ricci,
Fabio Poiesi
Computer Vision and Pattern Recognition (CVPR), Jun 2023 (github)
Method for novel class discovery for 3D point clouds based on online clustering exploiting uncertainty quantification to produce prototypes for pseudo-labelling points of novel classes.
|
|
The MONET dataset: Multimodal drone thermal dataset recorded in rural scenarios
Luigi Riz,
Andrea Caraffa,
Matteo Bortolon,
Mohamed Lamine Mekhalfi,
Davide Boscani,
Andre Moura,
Jose Antunes,
Andre Dias,
Hugo Silva,
Andreas Leonidou,
Christos Constantinides,
Christos Keleshis,
Dante Abate,
Fabio Poiesi
Computer Vision and Pattern Recognition Workshops (CVPRW), Jun 2023 (github)
MONET is a multimodal dataset captured using a thermal camera mounted on a drone that flew over rural areas, and recorded human and vehicle activities.
|
|
Overlap-guided Gaussian Mixture Models for Point Cloud Registration
Guofeng Mei,
Fabio Poiesi,
Cristiano Saltori,
Jian Zhang,
Elisa Ricci,
Nicu Sebe
Winter Conference on Applications of Computer Vision (WACV), Jan 2023 (github)
Overlap-guided probabilistic registration approach that computes the optimal transformation from matched Gaussian Mixture Model parameters.
|
|
Data Augmentation-free Unsupervised Learning for 3D Point Cloud Understanding
Guofeng Mei,
Cristiano Saltori,
Fabio Poiesi,
Jian Zhang,
Elisa Ricci,
Nicu Sebe,
Qiang Wu
British Machine Vision Conference (BMVC), Nov 2022 (github)
Augmentation-free unsupervised approach for point clouds to learn transferable point-level features via soft clustering.
|
|
CoSMix: Compositional semantic mix for domain adaptation in 3D LiDAR segmentation
Cristiano Saltori,
Fabio Galasso,
Giuseppe Fiameni,
Nicu Sebe,
Elisa Ricci,
Fabio Poiesi
European Conference on Computer Vision (ECCV), Oct 2022 (github)
Sample mixing for point cloud unsupervised domain adaptation, namely Compositional Semantic Mix (CoSMix), the first UDA approach for point cloud segmentation based on sample mixing.
|
|
GIPSO: Geometrically Informed Propagation for Online Adaptation in 3D LiDAR Segmentation
Cristiano Saltori,
Evgeny Krivosheev,
Stephane Lathuiliere,
Nicu Sebe,
Fabio Galasso,
Giuseppe Fiameni,
Elisa Ricci,
Fabio Poiesi
European Conference on Computer Vision (ECCV), Oct 2022 (github)
Adaptive self-training and geometric-feature propagation to adapt a pre-trained source model online without requiring either source data or target labels.
|
|
Distinctive 3D local deep descriptors
Davide Boscaini,
Fabio Poiesi
International Conference on Pattern Recognition (ICPR), Jan 2021 (github)
Learning distinctive 3D local deep descriptors (DIPs) that can be used to register point clouds without requiring an initial alignment.
|
|
Novel-View Human Action Synthesis
Mohamed I. Lakhal,
Davide Boscaini,
Fabio Poiesi,
Oswald Lanz,
Andrea Cavallaro
Asian Conference on Computer Vision (ACCV), Nov 2020 (project page)
Novel-View Human Action Synthesis aims to synthesize the movement of a body from a virtual viewpoint, given a video from a real viewpoint.
We employ 3D reasoning to synthesize the target viewpoint.
|
|
Seamless bare-hand interaction in Mixed Reality
Caterina Battisti,
Stefano Messelodi,
Fabio Poiesi
International Symposium on Mixed and Augmented Reality (ISMAR), Oct 2018 (video)
Unrealistic Mixed Reality (MR) experiences can be caused by unprocessed occlusions between real and augmented objects during interactions.
This can be addressed by blending real-time 3D finger tracking information with the visualisation of the hand in MR.
|
|
A distributed vision-based consensus model for aerial-robotic teams
Fabio Poiesi,
Andrea Cavallaro
Intelligent Robot and Systems (IROS), Oct 2018 (video)
Distributed model for a team of autonomous aerial robots to collaboratively track a target without external control.
The model uses distributed consensus to coordinate actions and to maintain formation via geometric constraints.
|
|
Cloud-based collaborative 3D reconstruction using smartphones
Fabio Poiesi,
Alex Locher,
Paul Chippendale,
Erica Nocerino,
Fabio Remondino,
Luc Van Gool
European Conference on Visual Media Production (CVMP), Dec 2017 (video)
Pipeline that enables multiple users to collaboratively acquire images with monocular smartphones and derive a 3D point cloud using a remote reconstruction server.
|
|
Online multi-target tracking with strong and weak detections
Ricardo Sanchez-Matilla,
Fabio Poiesi,
Andrea Cavallaro
European Conference on Computer Vision Workshops (ECCVW), Oct 2016
Online multi-target tracker that exploits both high- and low-confidence target detections in a Probability Hypothesis Density Particle Filter.
High-confidence detections are used for label propagation and target initialization.
Low-confidence detections only support tracking existing targets.
|
|
Detection of fast incoming objects with a moving camera
Fabio Poiesi,
Andrea Cavallaro
British Machine Vision Conference (BMVC), Sep 2016 (source code)
Moving object detection and avoidance algorithm for an uncalibrated camera that uses only the optical flow to predict collisions.
|
|
Distributed vision-based flying cameras to film a moving target
Fabio Poiesi,
Andrea Cavallaro
Intelligent Robot and Systems (IROS), Sep 2015 (video)
Infrastructure-free distributed control method for multiple flying cameras tracking a moving object.
Our vision-based servoing can deal with noisy and missing target observations, accounts for quadrotor oscillations and does not require an external positioning system.
|
|
Detection and tracking of groups in crowd
Riccardo Mazzon,
Fabio Poiesi,
Andrea Cavallaro
Advanced Video and Signal-Based Surveillance (AVSS), Aug 2013 (video)
Detecting and tracking interacting people by employing a framework based on a Social Force Model.
We model people approaching a group and restrict the group formation based on the relative velocity of candidate group members.
|
|
Detector-less ball localization using context and motion flow analysis
Fabio Poiesi,
Fahad Daniyal,
Andrea Cavallaro
International Conference on Image Processing (ICIP), Sep 2010 (video)
Estimating the location of the ball during a basketball game without using a detector.
We assume the ball is the point of focus of the game and that the motion flow of the players is dependent on its position during attack actions.
|
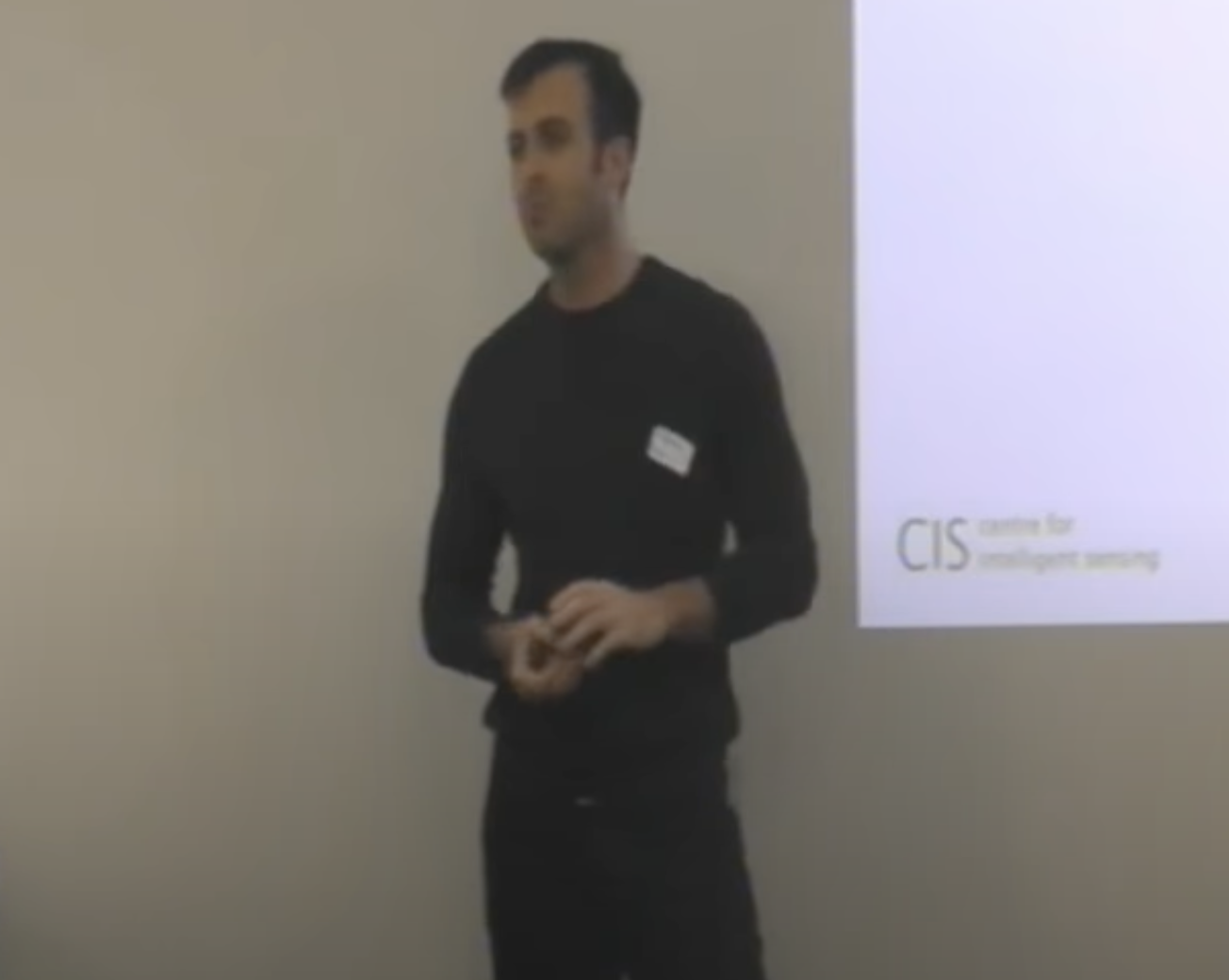 |
Point cloud deep descriptors and their application to machine perception (video)
Shandong University, Jinan, China, Sep 2023
Deep machine vision in digital industry (video)
Shandong University, Jinan, China, Nov 2022
Good practices learnt as a young PI (video)
University of Trento, Trento, Italy, May 2022
Learning general 3D descriptors for point cloud data
Istituto Italiano di Tecnologia, Genoa, Italy, Sep 2020
Localising, understanding and manoeuvring
Politecnico di Torino, Turin, Italy, May 2019
H2020 REPLICATE
Towards Photogrammetry 2020, Trento, Italy, Jun 2018
Formations of flying cameras to follow and film a moving target
Czech Technical University, Prague, Czech Republic, Mar 2016
Formations of flying cameras to film a moving target (video)
The London Big-O Meetup, Skills Matter, London, United Kingdom, Nov 2015
Multi-target tracking on confidence maps: an application to people tracking
University of Verona, Verona, Italy, Jun 2014
|
|